Public Medical Image Report Dataset to drive medical NLP research
There is no scarcity of research on the use of medical artificial intelligence for automatically generating reports from medical images such as simple X-ray scans, CT scans, or MRI scans. But, there exists no standard method for evaluating the quality of these critical medical reports. The reason for this is that the same medical result from a clinical scan may be written stylistically differently by different doctors. And, conversely, reports with different medical content may be stylistically similar. Furthermore, as standard practice in the medical field, only one reading report is created for an image, so simply collecting reading reports stored in medical institutions does not solve this problem as we don’t get sufficient information on how different doctors would write the same medical content.
In order to gather information on how medical contents are expressed, we asked multiple doctors to write a reading report for the same CT image(*1). The reports were created under a single criteria of “judging the stages of lung cancer from CT images by TNM classification (*2)”. The dataset thus created will help understand how diversely the medical contents of are expressed on medical image readings. A sample of the dataset is available on this site under the name of “Public Image Reading Report Dataset”, so please feel free to use it.
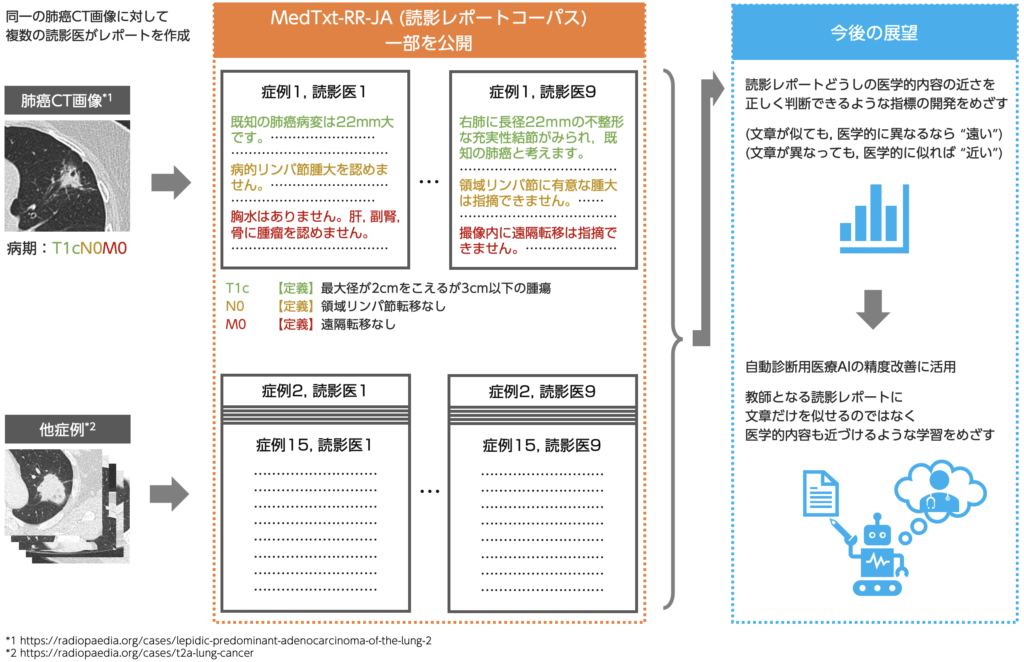
*1 We selected 15 lung cancer CT images that are available for free on Radiopaedia, and asked 9 reviewers to write review reports for each of them, collecting 135 documents in total..
*2 TNM classification: This is a widely used method to determine the progression (stage) of cancer. Three factors: the size of the cancer and the degree of invasion into surrounding organs (T factor), lymph node metastasis (N factor), and distant metastasis (M factor), are evaluated and the degree of cancer progression is determined by combining these factors. For example, a cancer diagnosis with a T factor of T1c, an N factor of N0, and an M factor of M0 would be classified as “T1cN0M0” in the TNM classification.
Download
- ReadingReports_202010 (updated:2020/10/08)
References
When you use MedTxt-RR, please cite:
Nakamura, Y, Hanaoka, S, Nomura, Y, Hayashi, N, Abe, O, Yada, S, Wakamiya, S, Aramaki, E. Clinical Comparable Corpus Describing the Same Subjects with Different Expressions. Stud Health Technol Inform, 290:253-257, 2022.
(BibTeX)
@Article{pmid35673012, Author="Nakamura, Yuta and Hanaoka, Shohei and Nomura, Yukihiro and Hayashi, Naoto and Abe, Osamu and Yada, Shuntaro and Wakamiya, Shoko and Aramaki, Eiji", Title="{{C}linical Comparable Corpus Describing the Same Subjects with Different Expressions}", Journal="Stud Health Technol Inform", Year="2022", Volume="290", Pages="253--257", Month="Jun" }
Privacy Policy
This is the privacy policy for the deliverables produced and published by Social Computing Laboratory, Nara Institute of Science and Technology. Please understand the contents of the product properly before using this software.
Disclaimer
This deliverable has been developed with the utmost care and a great attention to detail. However, reliability and robustness cannot be guaranteed completely and fully . We do not accept responsibility for any problems that may occur as a result of using this application or data. Please use it at your own risk.