- All OCR Extracted Text Documents (3148 documents) ←all non-text content removed by OCR error
- Frequency Balanced Subset (224documents) ←fixed word-level OCR errors, NER annotation included
If you would like to obtain this dataset, please contact our laboratory (email address below).
real-mednlp [at] is.naist.jp
The data is not available to the public on this site due to J-Stage’s terms of use and copyright regulations, but can be used free of charge if requested.
The details of each data are summarized below.
All OCR Extracted Text Documents
Dataset Creation
- Non-text removal: Visually detect and remove strings that cannot be detected as Japanese due to OCR errors
- Title, author name, header, footer, page numbers, references, figures, tables, captions, and English abstracts are also removed
- Sentence Formatting: Format the text to one sentence per line.
Format for the Data
A case report is filed in XML format with the following structure.
・・・Patient Info
For the case reports where the attribute value such as SEX or AGE is unknown, the value is assigned to “-1”.
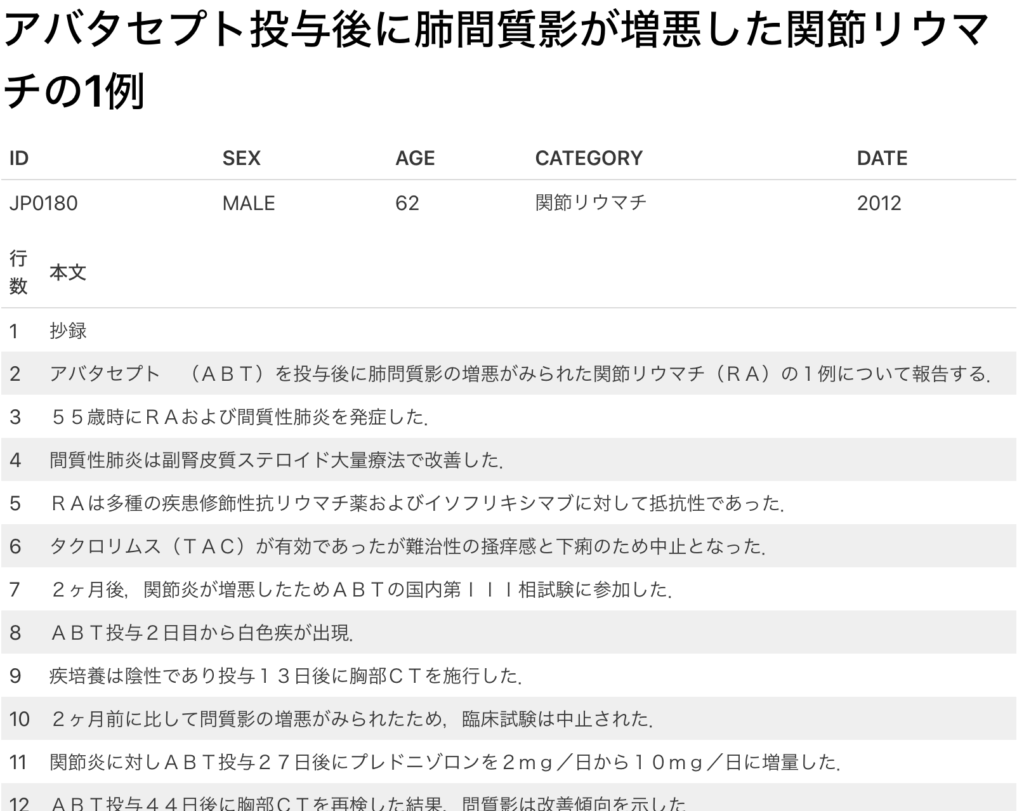
Example of a document (HTML formatted) — 和田 琢ほか「アバタセプト投与後に肺間質影が増悪した関節リウマチの1例」日本臨床免疫学会会誌, 35(5), 433-438, 2012.
Statistical Information
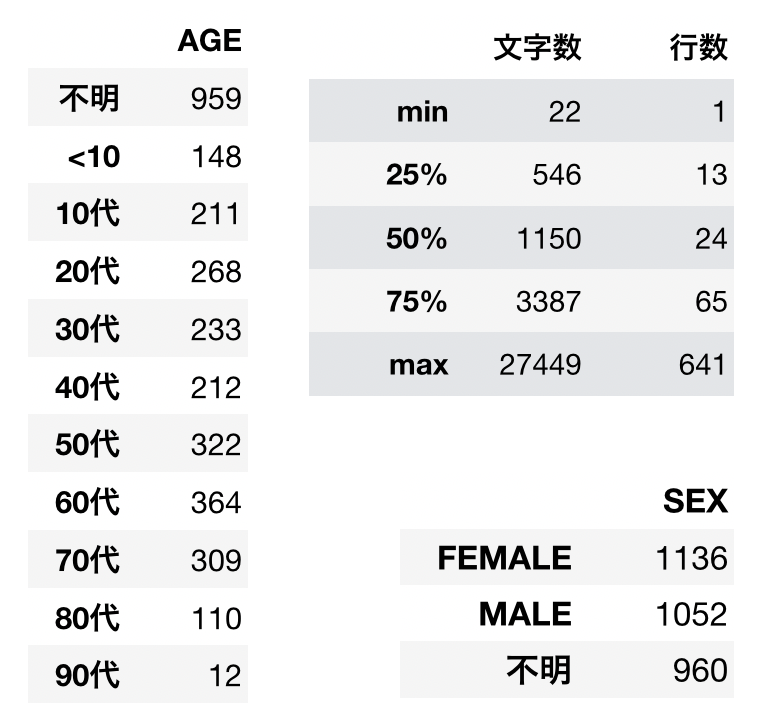
Frequency Balanced Dataset
Dataset Creation
- The number of documents has been balanced according to the actual frequency of occurrence for the common disease names.
- Other criteria:
- Maximum of 1,500 words in the text
- Reported cases should be relatively recent (published in and after 2010).
- Other criteria:
- Documents with many word/phrase level OCR errors are excluded.
- Word- and phrase-level OCR errors were corrected and recovered by viewing the PDF of the original article.
NER Annotation
The following medical expression entities were assigned as XML tags (tag names in parentheses).
- Disease (d)
- Anatomical part (a)
- Feature (f)
- Change (c)
- TIMEX3
- Test: [TestTest (t-test), TestKey (t-key), TestVal (t-val)]
- Medicine: [MedicineKey (m-key), MedicineVal (m-val)]
- Remedy (r)
- ClinicalContext (cc)
- Pending (p)
We used the the guidelines discussed in the following paper for the annotation.
Yada, S., Joh, A., Tanaka, R., Cheng, F., Aramaki, E., & Kurohashi, S. (2020). Towards a Versatile Medical-Annotation Guideline Feasible Without Heavy Medical Knowledge: Starting From Critical Lung Diseases. Proceedings of The 12th Language Resources and Evaluation Conference, 4567–4574.
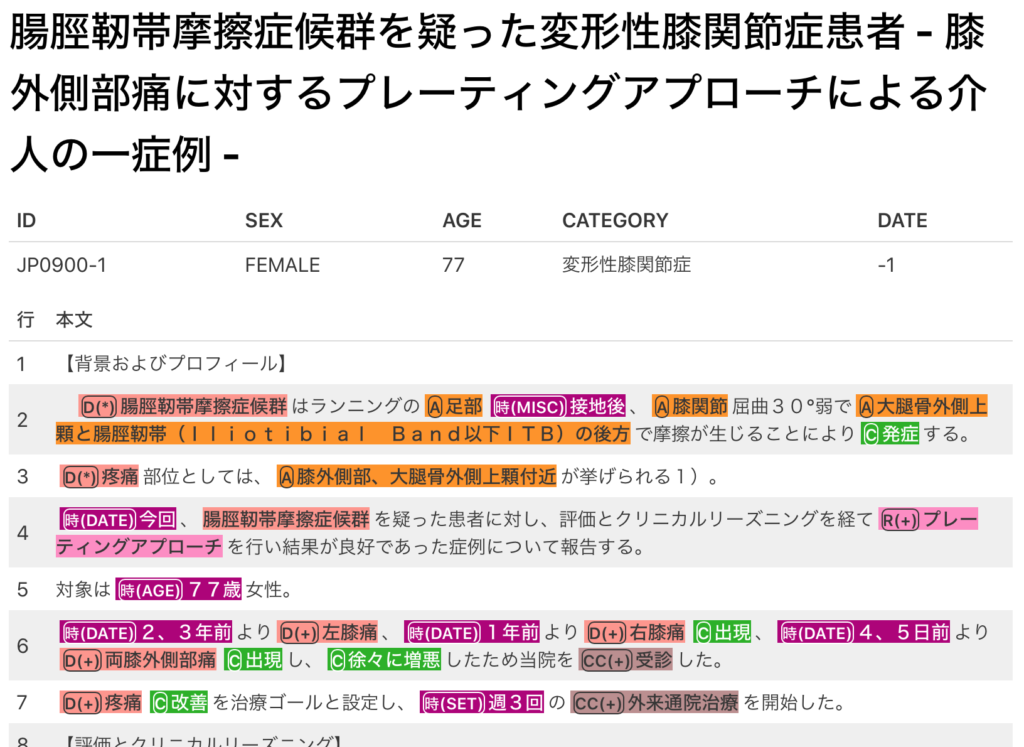
Statistical Information
(The following table refers to the past statistical count with 227 documents. The information is to be updated soon.)
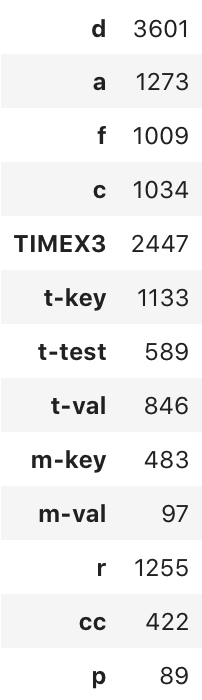
Total occurrences for each tag
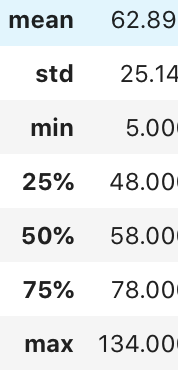
Statistical descriptions for tags included in a single document
References
Shuntaro Yada, Yuta Nakamura, Shoko Wakamiya, Eiji Aramaki: Real-MedNLP: Overview of REAL document-based MEDical Natural Language Processing Task, In Proceedings of the 16th NTCIR Conference on Evaluation of Information Access Technologies (NTCIR-16), pp. 285-296, 2022 [PDF]