News
- Jan 4, 2024: MedNLP-SC Social Media Corpus is publicly available.
- July 3, 2023: How to evaluate the SM subtask has been updated.
- June 28, 2023: The registration has closed.
- May 25, 2023: The registration deadline is extended to June 26, 2023.
- April 17, 2023: The number of data included in Social Media Corpus and MedTxt-RR Corpus has been updated, respectively.
- April 3, 2023: Training data are released.
- April 1, 2023: Sample data of Social Media Corpus are updated, and sample data of MedTxt-RR Corpus are available.
- March 23, 2023: The schedule is updated.
- March 10, 2023: Sample data of Social Media Corpus are available.
About MedNLP-SC
Medical Natural Language Processing for Social media and Clinical texts (MedNLP-SC) is one of the core tasks in NTCIR-17 for medical natural language processing using clinical texts written by physicians (radiology reports) or social media data. The goal of this shared task is to promote the development of practical systems that support various medical services.
The MedNLP-SC task has two corpus-based subtasks:
- Social Media (SM) Subtask (Adverse Drug Event detection for social media texts in Japanese, English, German, and French)
- Radiology Report (RR) Subtask (TNM staging for radiology reports in Japanese).
Datasets
Social Media Corpus
The data we provide for the challenge are artificially created tweets in several languages. We first generated Japanese tweets using a T5 model [1] fine-tuned on original Japanese Twitter messages. The generated tweets were then manually classified into two classes: those containing Adverse Drug Events (ADEs) vs. those not containing ADEs. Finally, our annotators labeled the occurring symptoms in the tweets containing ADEs. The most 22 frequent symptoms are “nausea,” diarrhea,” “fatigue,” “vomiting,” “loss of appetite,” “headache,” “fever,” “interstitial lung disease,” “liver damage,” “dizziness,” “pain,” “alopecia,” “analgesic asthma syndrome,” “renal impairment,” “hypersensitivity,” “insomnia,” “constipation,” “bone marrow dysfunction,” “abdominal pain,” “hemorrhagic cystitis,” “rash,” “stomatitis.” all other symptoms are collected below the class “other”. After annotation, the tweets were translated to English, German, and French using DeepL. The labels are the same for each language. For each language, we provide 9,957 tweets, divided into 80% training (7,964 tweets) and 20% test set (1,993 tweets). All drugs are represented in both sets except for one drug, which is only present in the test set. This is supposed to simulate the release of a new drug to the public.
[1] Colin Raffel, Noam Shazeer, Adam Roberts, Katherine Lee, Sharan Narang, Michael Matena, Yanqi Zhou, Wei Li, and Peter J. Liu. 2020. Exploring the limits of transfer learning with a unified text-to-text transformer. J. Mach. Learn. Res. 21, 1, Article 140 (January 2020), 67 pages.
JA アザチオプリンを服用して2ヶ月経ちました。1週間くらいで全身の発疹はなくなり、かゆみもほぼ無くなっていたのですが、麻疹が少し残ってて怖かったなぁと思います。
EN I’ve been on Azathioprine for 2 months now, and after about a week the rash all over my body was gone and the itching was almost gone, but I still had a bit of measles and I think it was scary.
DE Ich nehme jetzt seit zwei Monaten Azathioprin, und nach etwa einer Woche war der Ausschlag am ganzen Körper verschwunden und der Juckreiz fast weg, aber ich hatte immer noch ein bisschen Masern, und ich glaube, das war beängstigend.
FR Je prends de l’azathioprine depuis deux mois maintenant, et après environ une semaine, l’éruption cutanée sur tout mon corps avait disparu et les démangeaisons avaient presque disparu, mais j’avais encore un peu de rougeole et je pense que c’était effrayant.
Positive for “Rash”
JA アザチオプリン(イムラン)の副作用で脱毛がひどい。#潰瘍性大腸炎 <url>
EN Severe hair loss due to azathioprine (Imuran) side effects. #Ulcerative colitis <url>
DE Azathioprin (Imuran) Nebenwirkungen von schwerem Haarausfall. #Colitis ulcerosa <url>.
FR Effets secondaires de l’azathioprine (Imuran) sur la perte sévère de cheveux. #Colite ulcéreuse <url>.
Positive for “Alopecia”
JA
<user_name> で、アザチオプリンの血中濃度を調べてきました。やはりステロイド性肝障害が関係してるのかも?血液検査では炎症反応は上がっていたのですが、脱毛症状や発熱には至ってないようです。
EN <user_name> So I’ve been checking blood levels of azathioprine. Could it still be related to steroid-induced liver damage? The blood test showed an elevated inflammatory response, but it did not seem to lead to hair loss symptoms or fever.
DE <user_name> Also, ich habe die Blutwerte von Azathioprin überprüft. Könnte es immer noch mit einem steroidbedingten Leberschaden zusammenhängen? Der Bluttest zeigte eine erhöhte Entzündungsreaktion, aber es schien nicht zu Haarausfall-Symptomen oder Fieber zu führen.
FR <user_name> Donc, j’ai vérifié les niveaux sanguins d’azathioprine. Pourrait-il encore être lié à des lésions hépatiques induites par les stéroïdes ? L’analyse de sang a montré une réponse inflammatoire élevée, mais elle ne semble pas entraîner de symptômes de perte de cheveux ni de fièvre.
Positive for “Liver damage”
MedTxt-RR Corpus
A radiology report is a type of clinical document that is written by a radiologist. Basically, they focus on a single radiology image and describes all potential findings (including potential diseases) that can be expected from the image. While reports and target images are paired, most research on radiology reports tends to focus only on images, due to the hype surrounding image-based AI (such as automatic diagnosis of X-rays, CT, and MRI). One of the biggest problems when handling radiology reports is in the variety of writing styles. Although a diagnosis can be written in a variety of ways (diversity of expression), conventionally, only one report is created per image. As such, simply collecting reports from medical institutions may not yield enough information on the variability in reporting styles for the same diagnosis. Consequently, we included independent reports from multiple doctors for the same CT image.
The dataset comprises a set of 27 cases, in which 9 different radiologists describe the findings for each report. In total, 243 texts (126 texts for training and 117 texts for test) will be made available. We plan to make the dataset larger by recruiting additional cancer cases and radiologists.
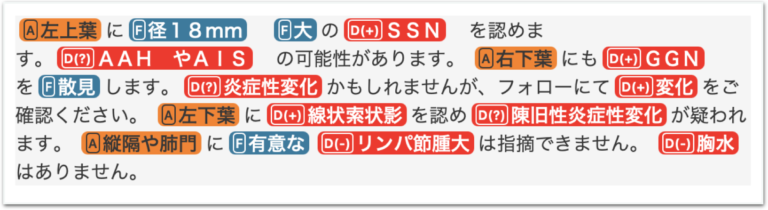
Task Overview
Social Media (SM) Subtask: Adverse drug event detection (ADE)
(Languages: Japanese, English, French, and German)
Task Definition: The participants can choose between the four different tracks Japanese, English, German and French. For each language, a separate system has to be submitted (it is also possible to submit the same system for several language tracks).
The task itself is divided into two parts:
- Classification of messages into “contains ADE (22 symptoms)” vs. “does not contain ADE (22 symptoms)”
- Multi-labeling of symptoms for all documents containing ADEs
Input: tweet
Output: symptom labels (either positive (1) or negative (0))
Examples: The examples below represent a simplified tweet. Only example ID1 gets a “positive (1)” label for the symptom “aspirin-induced headache” since it describes an adverse event. All other examples ID2-ID5 do not describe adverse events; therefore, all labels are set to “negative (0)”.
ID | text | headache | ... | stomatitis |
1 | I have an Aspirin-induced headache. | 1 | 0 | 0 |
2 | I have a headache which I am treating with Aspirin. | 0 | 0 | 0 |
3 | I don't have an Aspirin-induced headache. | 0 | 0 | 0 |
4 | I have a headache. | 0 | 0 | 0 |
5 | I found an article on Aspirin-induced headache. | 0 | 0 | 0 |
Evaluation: For evaluation, the data was split into 80% training samples and 20% test samples. Since there are 22 classes an ADE-positive sample can belong to, finding exact matches can be difficult. Therefore, we evaluate the system predictions in four ways:
- Binary: A sample is considered to contain an ADE if at least one symptom (class) is positive (1). This metric calculates the performance (precision, recall, and F1 score) of classifying a sample into the classes “contains ADE” (positive) vs. “does not contain ADE” (negative).
- Per class (symptom): Calculates precision, recall, and F1 score for each class (symptom) individually and averages over classes.
- (Full) per label: Calculates precision, recall, and F1 score for each label (0 and 1) across samples and classes.
- Exact match accuracy: Calculates the percentage of perfect matches.
Radiology Report (RR) Subtask: TNM staging
(Language: Japanese)
This is a MedNLP-SC original task to evaluate the generalization ability of NLP models when classifying radiology reports under multiple criteria. Focusing on cancer staging, task participants are required to assign four labels, tumor (T), lymph node (N), metastasis (M), and clinical stage (cStage) for each report according to predefined criteria. Early cancers will be labeled with small numbers such as T1N0M0, cStage 1, and advanced cancers will be labeled with large numbers such as T3N2M1, cStage 4. Cancer staging is essential for treatment and research, but it requires complex reasoning. We aim to explore the capability of NLP for automated cancer staging to aid clinical professionals.
Schedule
March 2023: Dataset release(March-June 2023: Dry run)June 1June 26, 2023: Registration deadlineJune 26, 2023: Training data (final version) releaseJuly 10, 2023: Test data releaseJuly 17, 2023: Result submission- August 1, 2023: Evaluation result release
- August 1, 2023: Task overview paper release (draft)
- September 1, 2023: Submission due of participant papers (draft)
- November 1, 2023: Camera-ready participant paper due
- December 12-15, 2023 (JST): NTCIR-17 Conference in NII, Tokyo (Online presentation will be available)
Note: All are in the AoE time zone except for the conference schedule.
Entry Form
Organizer
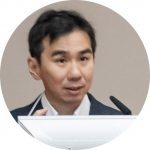
Co-chair (general)
Eiji Aramaki, Ph.D. (NAIST, Japan)
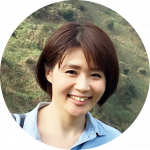
Co-chair (general)
Shoko Wakamiya, Ph.D. (NAIST, Japan)
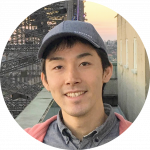
Co-chair (SM Subtask)
Shuntaro Yada, Ph.D. (NAIST, Japan)
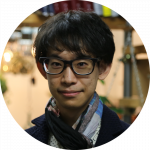
Co-chair (RR Subtask)
Yuta Nakamura, M.D. (The University of Tokyo, Japan)
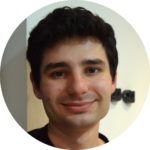
SM Subtask
Gabriel Herman Bernardim Andrade (NAIST, Japan)
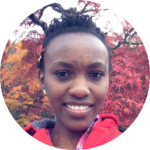
SM Subtask
Faith Wavinya Mutinda, Ph.D. (NAIST, Japan)
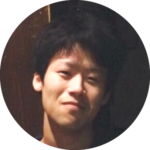
SM Subtask
Tomohiro Nishiyama (NAIST, Japan)
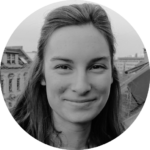
SM Subtask
Lisa Raithel (DFKI, Germany, TU Berlin, Germany, and Université Paris-Saclay, CNRS, LISN, France)
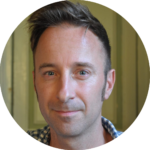
SM Subtask
Roland Roller, Ph.D. (DFKI, Germany)
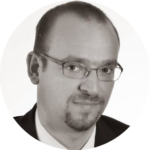
SM Subtask
Philippe Thomas, Ph.D. (DFKI, Germany)
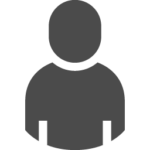
SM Subtask
Cyril Grouin, Ph.D. (Université Paris-Saclay, CNRS, LISN, France)
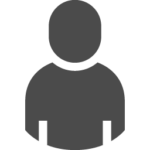
SM Subtask
Thomas Lavergne, Ph.D. (Université Paris-Saclay, CNRS, LISN, France)
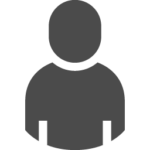
SM Subtask
Aurélie Névéol, Ph.D. (Université Paris-Saclay, CNRS, LISN, France)
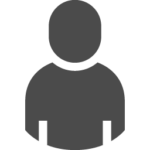
SM Subtask
Patrick Paroubek, Ph.D. (Université Paris-Saclay, CNRS, LISN, France)
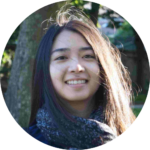
SM Subtask
Hui-Syuan Yeh (Université Paris-Saclay, CNRS, LISN, France)
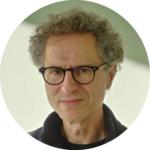
SM Subtask
Pierre Zweigenbaum, Ph.D. (Université Paris-Saclay, CNRS, LISN, France)
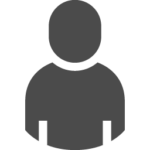
SM Subtask
Akiko Aizawa, Ph.D. (NII, Japan)
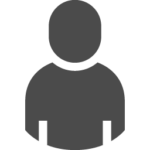
RR Subtask
Shouhei Hanaoka, M.D., Ph.D. (The University of Tokyo, Japan)
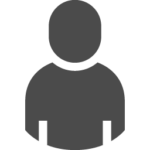
SM Subtask
Yuji Matsumoto, Ph.D. (RIKEN, Japan)
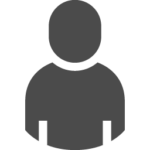
SM Subtask
Noriki Nishida, Ph.D. (RIKEN, Japan)
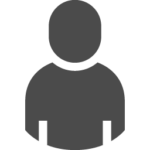
SM Subtask
Hiroki Teranishi, Ph.D. (RIKEN, Japan)
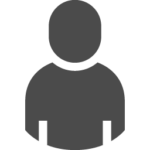
SM Subtask
Narumi Tokunaga (RIKEN, Japan)
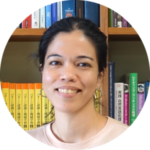
SM Subtask
Lis Weiji Kanashiro Pereira Ph.D. (NAIST, Japan)
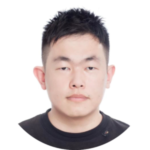
SM Subtask
Peitao Han (NAIST, Japan)
Collaborators
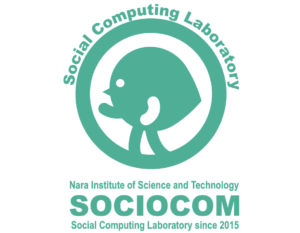
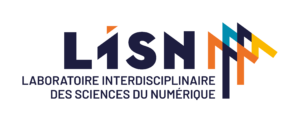
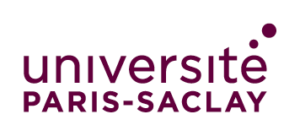
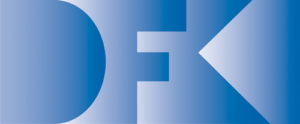
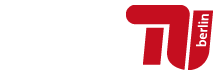
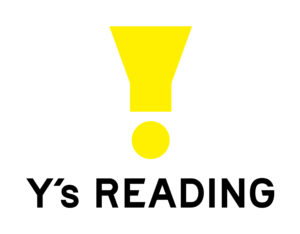
Acknowledgements
Social Media (SM) Subtask is supported by KEEPHA project of JST, AIP Trilateral AI Research, Grant Number JPMJCR20G9, Japan. Radiology Report (RR) Subtask is supported by JST CREST Grant Number: JPMJCR22N1, JST AIP-PRISM, and MHLW Program Grant Number JPMH21AC500111, Japan.
Inquiry
- MedNLP-SC office email: mednlp-sc[at]is.naist.jp