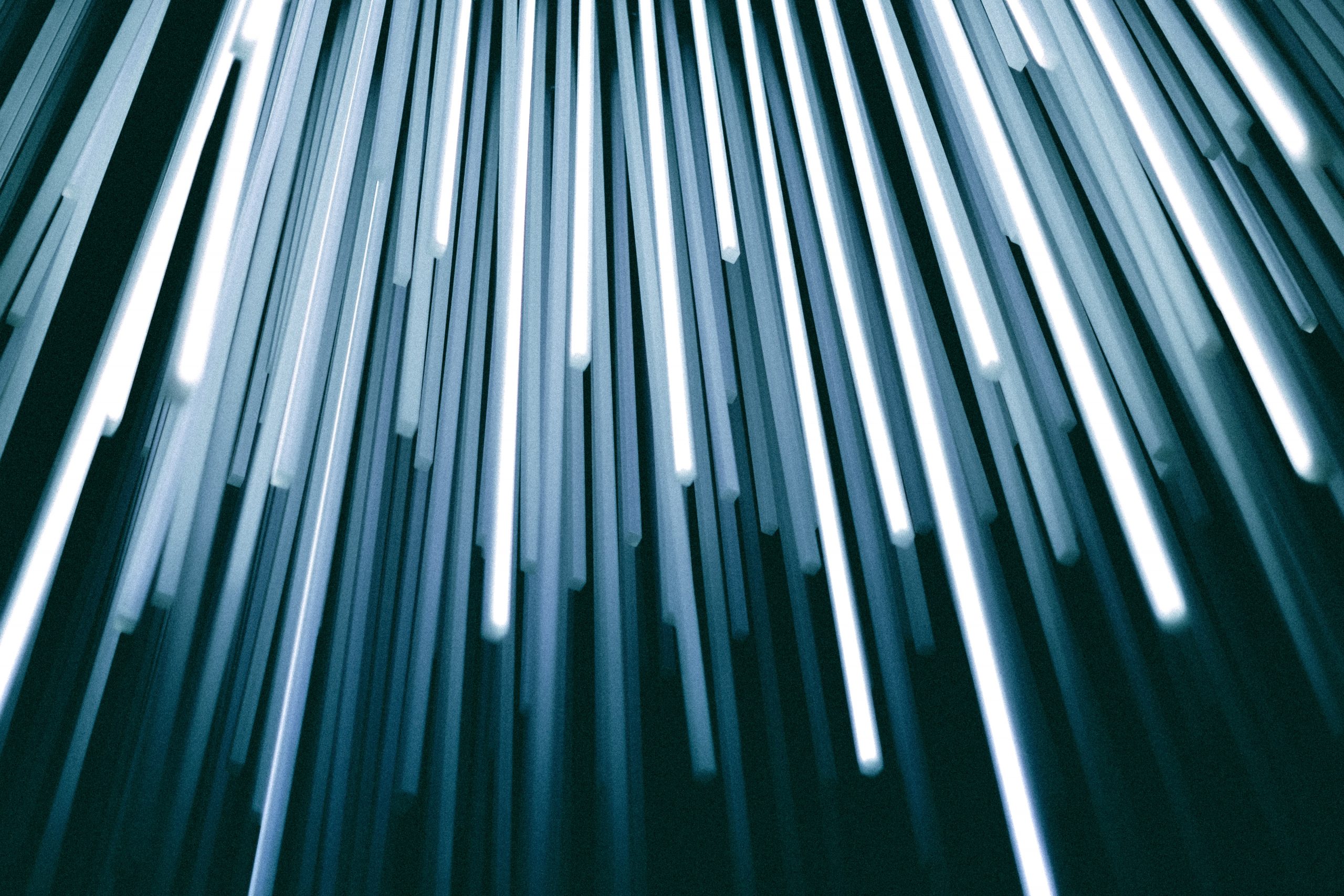
NTCIR-16 Real-MedNLP
With the advent of the internet age, medical records are increasingly being written in electronic formats in place of paper, which leads to a higher importance of information processing techniques in medical fields. However, the amount of privacy-free medical text data is still small in non-English languages, such as Japanese and Chinese. In such a situation, we had proposed a series of previous four medical natural language processing (MedNLP) tasks, MedNLP-1, MedNLP-2, MedNLPDoc, and MedWeb. This task will yield promising technologies to develop practical computational systems for supporting a wide range of medical services.
NEWS
About this workshop
Real-MedNLP is a shared task workshop for medical language processing using actual medical documents (case reports and radiology reports). The goal of this task is to promote the development of practical systems that support various medical services.
The Real-MedNLP task has two corpus-based tracks (MedTxt-CR Track and MedTxt-RR Track), each with three subtasks.
The task overview paper is available here.
Datasets
MedTxt-CR Corpus
This dataset comprises a set of open-access case reports available at CiNii. Typically, open-access case reports are biased in the reporting of patients and diseases, due to differences in the policies various medical societies have towards open-access publications.
To reduce such bias caused by each medical society’s publication policy, we select case reports based on actual frequencies of patients and diseases.
- Training set: 100 reports
- Test set: 100 reports
A case report is detailed descriptions of a patient’s medical condition for research purposes. They track the onset and temporal progression of the patient’s disease and are large in quantity as medical societies typically have dedicated submission tracks for consolidating these reports. Considering these advantages, case reports possess enormous potential as a rich source of information. Furthermore, the format of a case report is similar to that of a discharge summary, which is frequently used in healthcare contexts. Techniques developed here for case report analysis could also be applied to analyze discharge summaries.
MedTxt-RR Corpus
This dataset comprises a set of 15 cases, in which 9 different radiologists describe the findings for each report. In total, 135 texts will be made available.
- Training set: 72 texts
- Test set: 63 texts
A radiology report is a type of clinical document that is written by a radiologist. Basically, they focus on a single radiology image and describes all potential findings (including potential diseases) that can be expected from the image. While reports and target images are paired, most research on radiology reports tends to focus only on images, due to the hype surrounding image-based AI (such as automatic diagnosis of X-rays, CT, and MRI). One of the biggest problems when handling radiology reports is in the variety of writing styles. Although a diagnosis can be written in a variety of ways (diversity of expression), conventionally, only one report is created per image. As such, simply collecting reports from medical institutions may not yield enough information on the variability in reporting styles for the same diagnosis. Consequently, we included independent reports from multiple doctors for the same CT image.
Task Overview
Few-resource Named Entity Recognition (NER)
Since NER is arguably the most fundamental information extraction problem for MedNLP, we designed challenges pertaining to NER on our real-world clinical documents, with a sample of only 100-200 documents. This scale of corpus size tends to be regarded as a “few-resource machine learning”, which is a de-facto standard for any sort of MedNLP in general.
Subtask 1: Just 100 Training
- NER using the training set consisting of 100 documents
- This subtask is equivalent to standard supervised learning with few resources
Subtask 2: Guideline Learning
- NER using the example sentence(s) for each tag in Annotation Guideline and not using the training set for Subtask 1
- This simulates the training of human annotators, who often learn from the annotation guidelines provided by researchers.
<article id="JP0217-29" title="著明な好酸球増多を伴った非昏睡型急性肝不全の一例"> Case Study: <TIMEX3 type="AGE">53 year old</TIMEX3> female patient. Chief Complaint: <d certainty="positive">Fever</d>. Progress: Patient was <cc state="executed">seen</cc> at the dermatology department of our hospital <TIMEX3 type="DATE">2 years before 20XX</TIMEX3> and presented a <d certainty="positive">skin rash</d> that the diagnosis identified as <d certainty="positive">bullous pemphigoid</d>. <m-key state="executed">Prednisolone (PSL)</m-key> <m-val>1 mg/kg/day</m-val> was introduced and the patient <TIMEX3 type="TIME">was</TIMEX3> managed with concomitant <m-key state="executed">immunomodulators</m-key> with <c>a gradual decrease</c> in the <m-key state="executed">PSL</m-key> level. <m-key state="negated">PSL</m-key> was voluntarily discontinued in <TIMEX3 type="DATE">August, 20XX</TIMEX3> when the <m-key state="executed">PSL</m-key> dosage had been <c>reduced</c> to <m-val>6 mg/day</m-val>, but there was no <d certainty="negative">worsening of the skin rash</d>. ...(skipped)... </article>
[Updated on October 22, 2021] Irrelevant pages were removed from the Annotation Guidelines
[Updated on December 20, 2021] A small portion of text and annotation examples were fixed
Application
Subtask 3: Application
[MedTxt-CR Track] Adverse Drug Event detection (ADE)
Extract adverse drug event (ADE) information from case reports and fill out a table
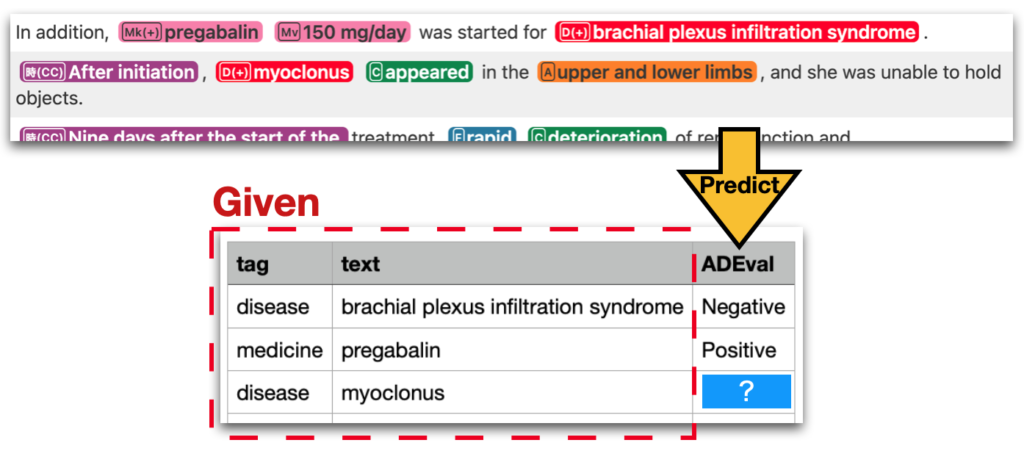
[MedTxt-RR Track] Case Identification (CI)
Identify the radiology reports for the same case
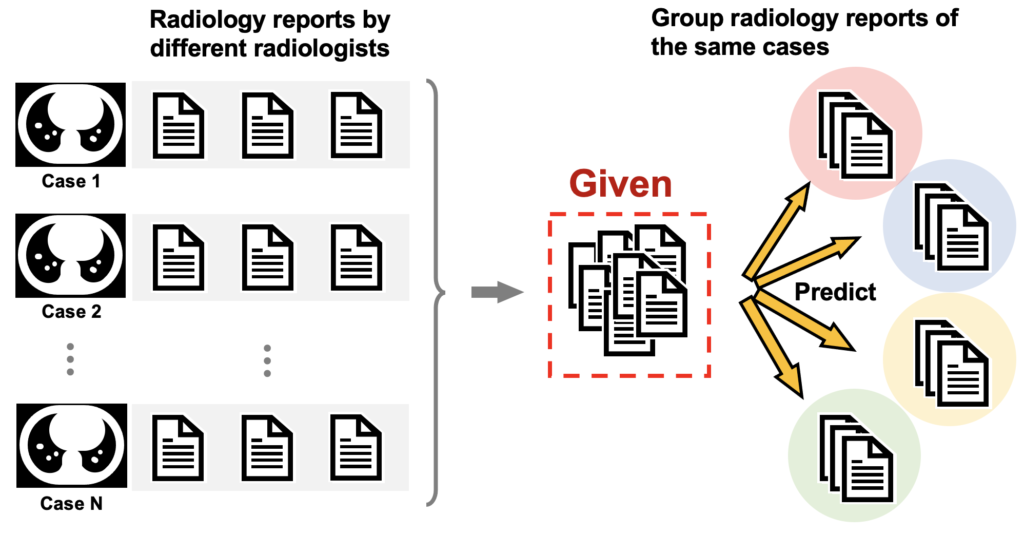
Schedule
September 30, 2021: Datasets release (email to registered teams)September 1 December 1, 2021: Registration DueJanuary 10-17, 2022: Formal run of Subtasks 1 and 2January 10, 2022: Test data release (the organizer to participants)January 17, 2022: Result submission (participants to the organizer)
January 18-25, 2022: Formal run of Subtask 3 ADE and CIJanuary 18, 2022: Test data release (the organizer to participants)January 25, 2022: Result submission (participants to the organizer)
February 1, 2022: Evaluation Result ReleaseFebruary 1, 2022: Draft Task Overview Paper ReleaseMarch 1, 2022: Draft Participant Paper Submission DueMay 1, 2022: All Camera-ready Paper Submission DueJune 14-17, 2022: NTCIR-16 Conference (Online) [Program] [Registration]June 17, 2022: Real-MedNLP Session
FAQ
In Subtask 1, participants can use the training dataset to supervise your model. In Subtask 2, participants cannot use the training dataset to supervise a model, whereas participants may use the example sentences appearing in the annotation guidelines (as if a model is a human annotator).
Both subtasks allow participants to include any external resource (i.e., semi-supervision is OK).
Clear reports (removed all tags) are provided as the test dataset. Participants should add tags to the test data like the training data.
In Subtasks 1 and 2, we plan to perform two-level evaluations: the entity level and the entity+attribute level (joint). In the joint evaluation, it is regarded as correct if both the entity and the attribute match, and incorrect if the attribute matches but the entity does not. We recommend to recognize both the entity and the attribute from a practical perspective, though whether or not to deal with the attribute is optional.
We plan to employ various evaluation metrics for Subtasks 1 and 2 such as precision, recall, F1 (micro, macro). The details will be announced later.
No, it does not include the NER task in the ADE challenge. We provide the reports with tags for the ADE challenge. Since “articleID,” “tag,” and “text” are given, participants should answer the value of “ADEval.”
Since the CI challenge is a clustering task, we evaluate the performance with Normalized Mutual Information (NMI).
The formal run period would be about one week just after the test set release.
The schedules of the three subtasks are the same, excepting the ADE challenge in Subtask 3, which uses the results of NER (Subtasks 1 and 2) in the formal run. Therefore, the test set for the ADE challenge of Subtask 3 will release after the formal run of Subtasks 1 and 2. That is, the flow in the formal run is as follows: Release of the test set for Subtasks 1 and 2 -> The formal run of Subtasks 1 and 2 (for a week) -> Release of the test set for Subtask 3 -> The formal run of Subtask 3 (for a week).
No, we plan to evaluate the following tag sets for Subtasks 1 and 2:
CR: <d>, <a>, <timex3>, <t-test>, <t-key>, <t-val>, <m-key>, and <m-val>
RR: <d>, <a>, <timex3>, and <t-test>
Organizer
- Eiji Aramaki (Nara Institute of Science and Technology, Japan)
- Shoko Wakamiya (Nara Institute of Science and Technology, Japan)
- Shuntaro Yada (Nara Institute of Science and Technology, Japan)
- Yuta Nakamura (The University of Tokyo, Japan)
Collaborators
Acknowledgements
MedTxt-RR Track and annotation were supported by JST AIP-PRISM, Japan. MedTxt-CR Track was supported by KEEPHA project of JST, AIP Trilateral AI Research, Grant Number JPMJCR20G9, Japan.
Call for Sponsors
Real-MedNLP is looking for sponsors. If you are interested in sponsoring Real-MedNLP, please contact us by e-mail.
Sponsors are also welcome to participate in the shared task.
Inquiry
- Real-MedNLP office email: real-mednlp[at]is.naist.jp
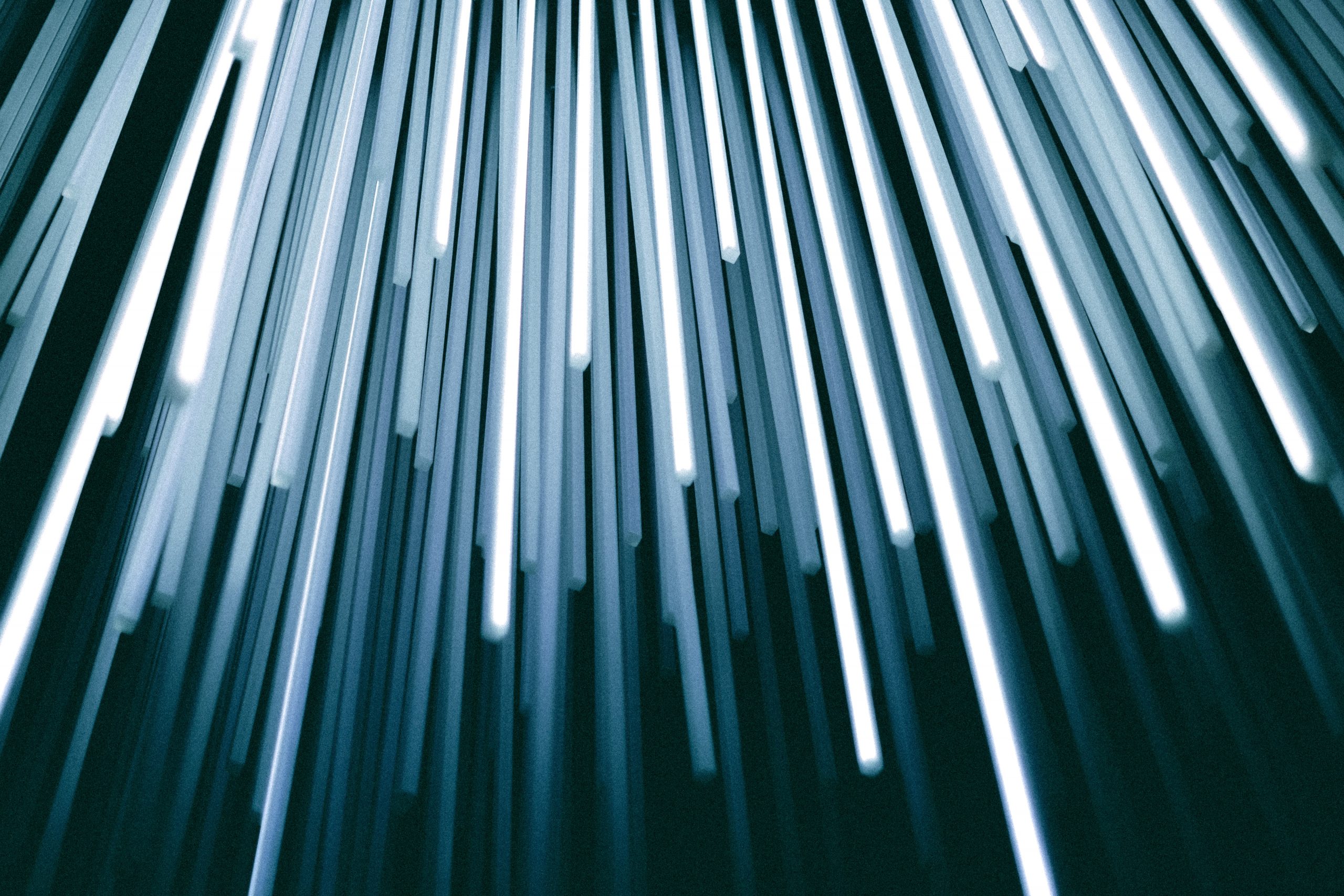